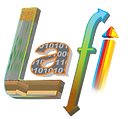
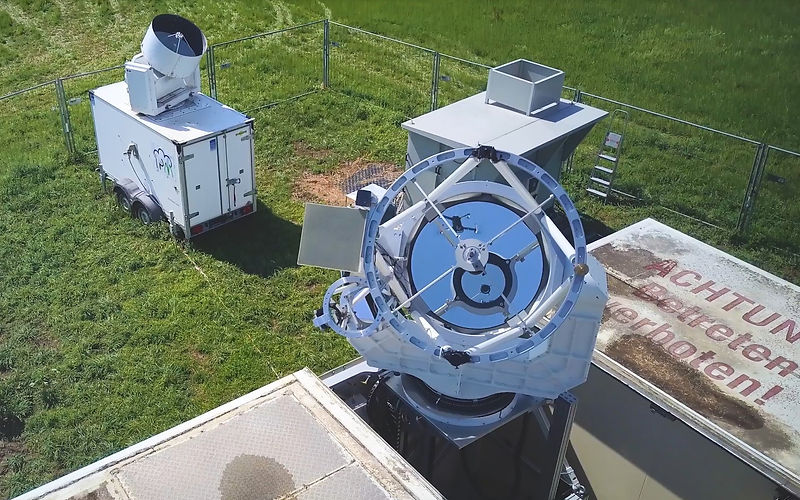
Measurements
To advance the understanding of Land-Atmosphere processes and the resulting local and non-local feedbacks, observations in all compartments of the L-A System with high vertical and horizontal resolutions need to be combined. For this purpose, the instrumentation at the Land-Atmosphere Feedback Observatory (LAFO) will be expanded by a sophisticated sensor synergy which will address all objectives of LAFI. This requires a joint operation of scanning lidar systems, FODS, UAVs, and in-situ sensors for observing (partitioning) ET and many other soil, plant and atmospheric quantities. The vertical and horizontal structures of key variables in the L-A system will be observed simultaneously to capture the influence of land surface heterogeneities, such as micro- to mesoscale circulations, on L-A feedbacks.
The envisioned experimental design is depicted in Fig. 1. The observations will be performed within a General Operations Period (GOP) into which a series of five Intensive Observations Periods (IOPs) are embedded. During the GOP (2nd LAFI year), the operational instrumentation will run during a full vegetation period (early spring till late autumn).
​
​
​
​
​
​
​
​
​
​
​
​
​
​
​
Fig. 1: Overview of LAFO.
The majority of the measurements will focus on four plots, where maize and winter wheat are grown (fields 1 and 2 on Fig. 2), to study two of the most important crops in Europe and the land surface heterogeneities induced by agricultural land use. Spaceborne remote sensing will complement these observations by providing information on heterogeneity of soil and land cover over a larger domain.
​
​
​
​
​
​
​
​
​
Fig. 2: Experimenatal design of the LAFO GOP and IOPs.
The five IOPs will take place in five critical phenological periods, during which the entire LAFI sensor synergy will be employed: (1) stem elongation (BBCH 30-39) of wheat with maximum water demand in April; (2) booting (BBCH 40-49) of wheat in May; (3) flowering (BBCH 60-69) of wheat and beginning leaf development (BBCH 10-19) of maize in June; (4) ripening (BBCH 80-89) of wheat and continued leaf development of maize in July; (5) flowering of maize with maximum water demand in August. The exact IOP times and lengths will be kept flexible and governed by observed meteorological conditions and vegetation development.
Furthermore, to enhance our understanding of fluxes at the land surface and their profile in the ABL including entrainment, and the statistical analysis of extreme events, the long-term measurements of the Meteorological Observatory Lindenberg - Richard Aßmann Observatory (MOL-RAO) are analyzed.
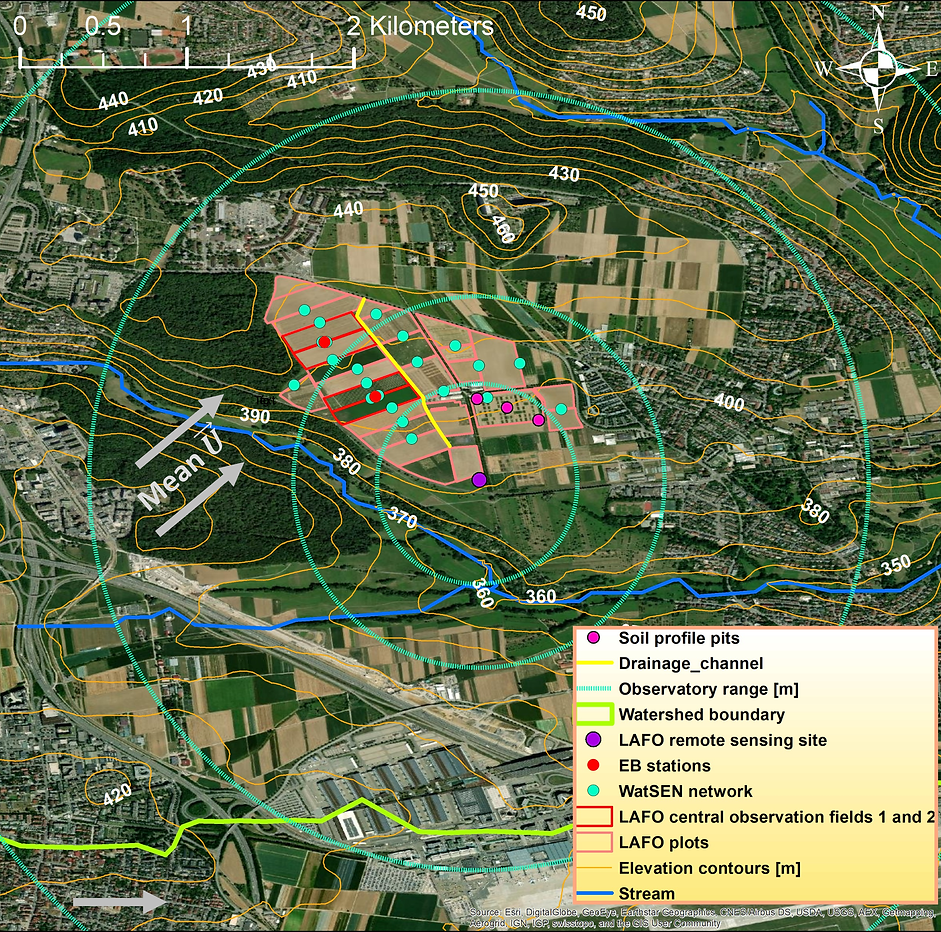

Modeling
In order to quantify the impacts of non-local processes on measured quantities in the local L-A system, modeling systems are required. Models can operate on different spatial and temporal scales and simulate processes from the micro-gamma to the meso-gamma scale and their respective interrelations, thus enabling a comprehensive analysis of the L-A system.
However, to derive robust results from such model simulations, it is of fundamental importance that the simulations are performed with different modeling systems, because every modeling systems is associated with uncertainties. Therefore, the LAFI simulations encompass the weather and climate models WRF and ICON, and the turbulence-resolving large-eddy simulation model PALM. These atmospheric models are coupled to different Land Surface Models in order to specifically consider the uncertainties associated with the interactions and exchange processes between the soil, the vegetation and the lowest part of the atmospheric boundary layer. These modeling systems form together the LAFI Multi Model Experiment (MME).
The nesting chain of the LAFI MME is shown in Fig. 3. The simulations for the outer model domain are conducted on the convection-permitting scale (1.25 km). In a first step, long-term simulations over the general observation period (GOP) in LAFI are performed with WRF-NoahMP and ICON on this domain. On top of this, additional WRF-NoahMP and ICON-LEM simulations are performed in a further increased spatial resolution for the LAFI intense observation periods (IOPs). Because of its high computational costs, PALM simulations are only performed for the IOPs.
​
​
​
​
​
​
​
​
Fig. 3: Nesting chain for the GOP (outer domain) and the IOPs (inner domains) for LAFO (upper row) and MOL-RAO (lower row).
​
By means of such a modeling strategy, it can be guaranteed that meso-scale atmospheric conditions are considered, and that local processes around the LAFI measurement sites are simulated in the required spatial and temporal resolution, for both, the IOPs and the GOP. Thus, a comparison of observations and model runs is guaranteed over the whole LAFI measurement period.
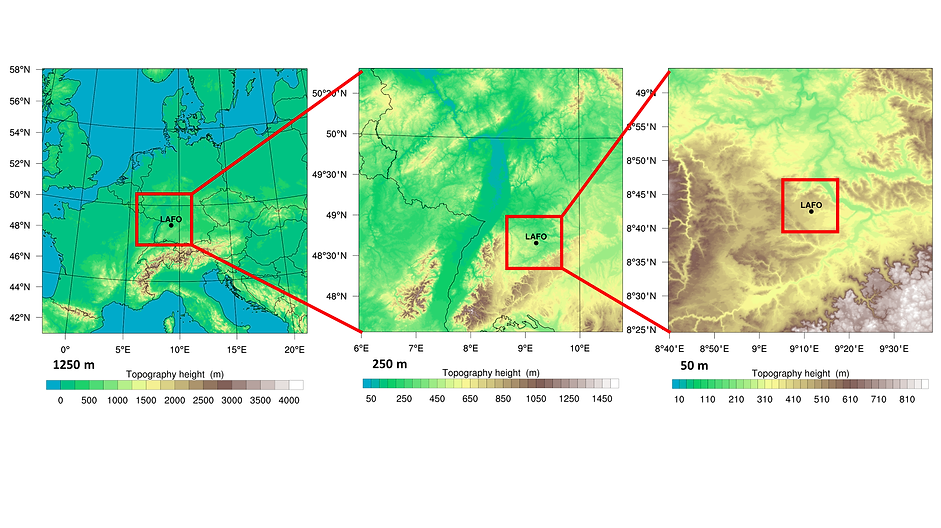
Deep Learning
The LAFI consortium will generate large amounts of observations and model outputs of the L-A system with unprecedented detail and resolutions. However, understanding the processes and feedbacks underlying this complex data is a very challenging task. The atmospheric flow is chaotic in nature. The actual interactive complexity of turbulence dynamics within the atmospheric boundary layer and entrainment fluxes is still poorly understood. The highly non-linear interaction between many variables of the L-A system is therefore very difficult to describe.
In recent years, however, with deep learning (DL) techniques, methods were developed which are specifically designed to identify hidden internal structures from large and complex datasets. In the framework of LAFI, these capabilities of DL will be exploited to extract the systematic interrelations underlying the processes and feedbacks in the L-A system. More precisely, we will 1) develop hierarchies of machine learning approaches and apply importance weighting to identify key driving parameters and variables; 2) enhance DL and other machine learning techniques with physics-informed mechanisms; 3) include L-A system-informed inductive biases in DL, and 4) develop DL-based foundation models, which will target the inference of general, L-A state characterizing structures. In this connection, we aim not only for improved representations of relationships or identification of structures but also for an improvement of process understanding.
Fig. 4: Schematic of a foundation model
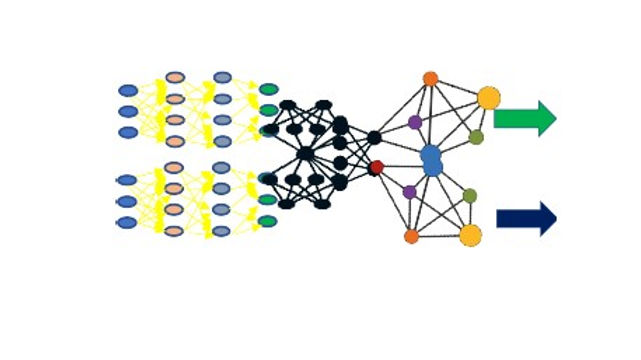